Starting a New Job
You can start the process of enlisting the help of our External Labelling Service by entering your project and accessing the Annotator page. Once you are there, a popup card will appear that will invite you to consider hiring labellers. If you agree, an onboarding display will show up with four steps.

Step 1: Design a Brief
The first step, ‘Design a Brief’ requests that you give a brief description of your project and describes what types of objects you seek to annotate and what annotation type you require. Other inputs that are required are an approximate budget that you would be willing to use for annotation, as well as at least 8 annotated images on the project. The purpose of the 8 annotated images is to provide sample quality and helps to identify objects that are not commonly known or use case specific for the hired labellers. Ideally, the annotated images should be as representative of your dataset as possible. They should show example annotations of all the different types of objects as well as aptly demonstrate the required detail and precision.
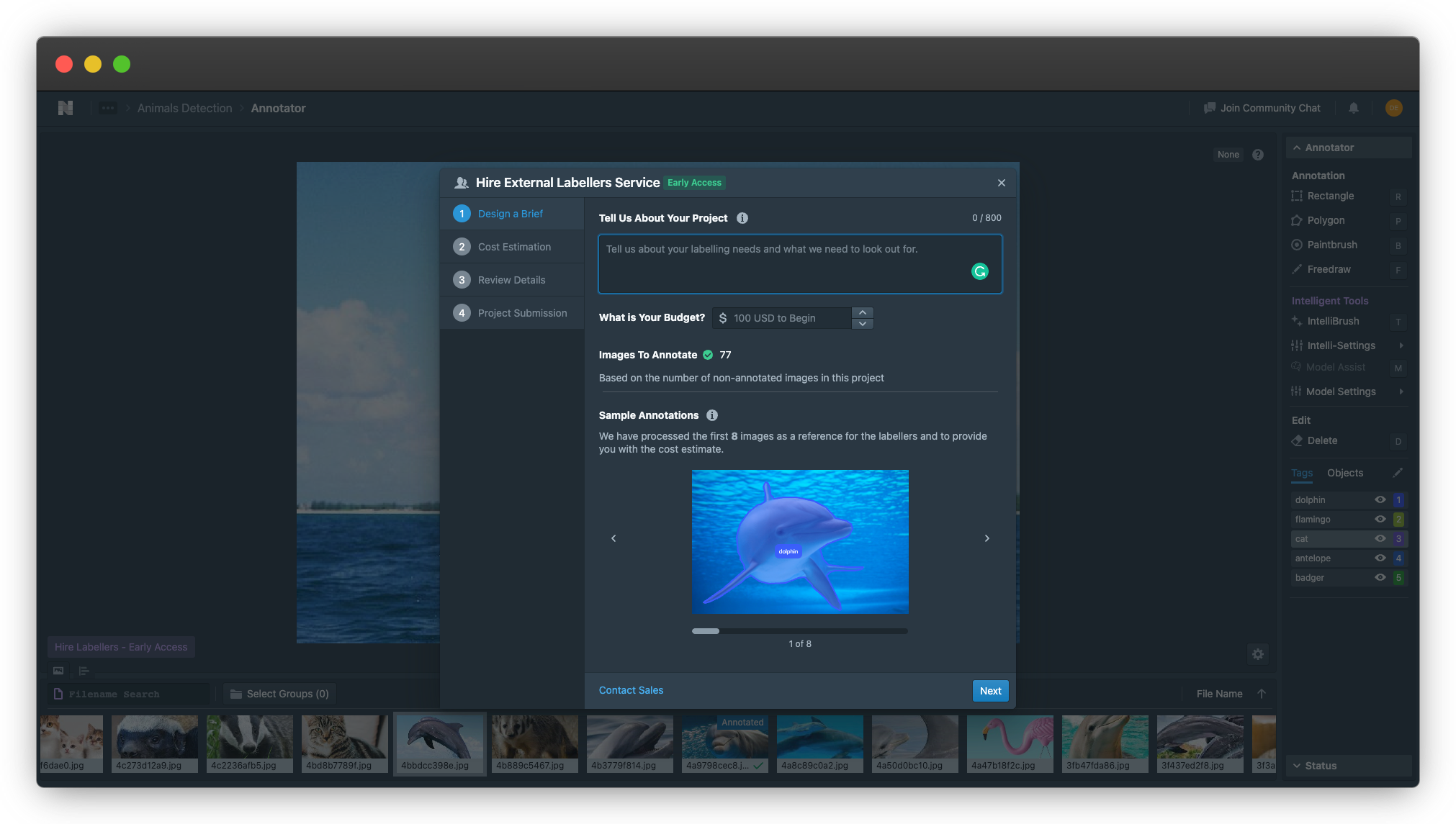
Step 2: Cost Estimation
The second step defines the specific requirements of the annotation task. Here, you can choose between three options in terms of annotating pace: ‘Basic’, ‘Standard’, and ‘Priority’. The different options vary in terms of how many annotators are assigned to the project. The slider below is used to allow you to select how many images you want to have annotated. At the very bottom is the estimated cost calculated based on the options you have inputted here.
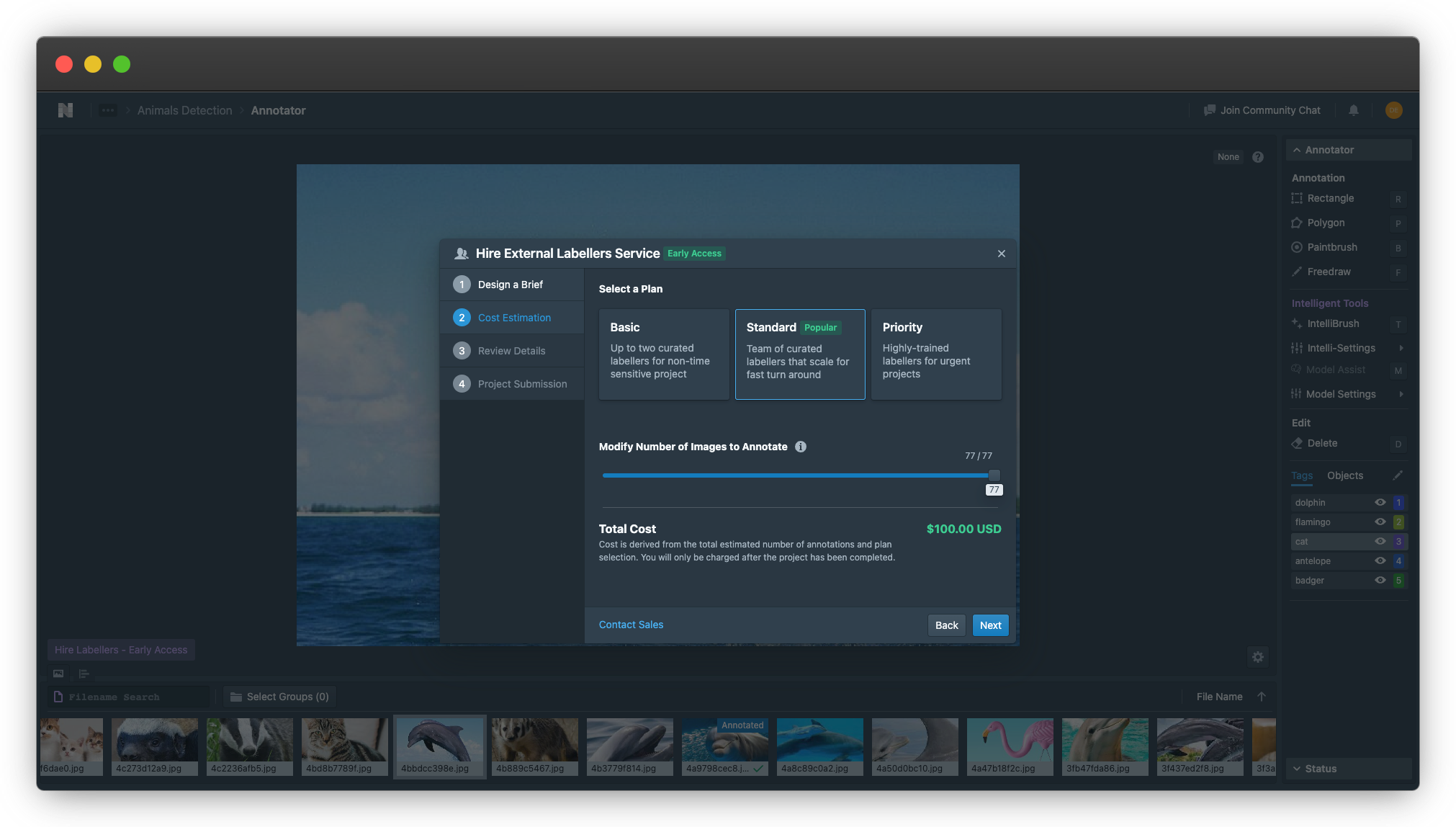
Step 3: Review Submission
Once you are happy with your options, you will be brought to the review page, where you will be displayed your project description, the number of images that have to be annotated, the pace at which you would like the annotations to be done at, and finally, the estimated total cost. To progress and send in the request, you have to agree to the terms and conditions which are shown in another tab next to the project summary. If you have any changes that you would like to have made on previous inputs, you can always return to previous steps. Once your request has been made, the request will automatically be transferred to us, who will then file a formal request with our external labelling partners. An email will be sent as soon as all is confirmed.
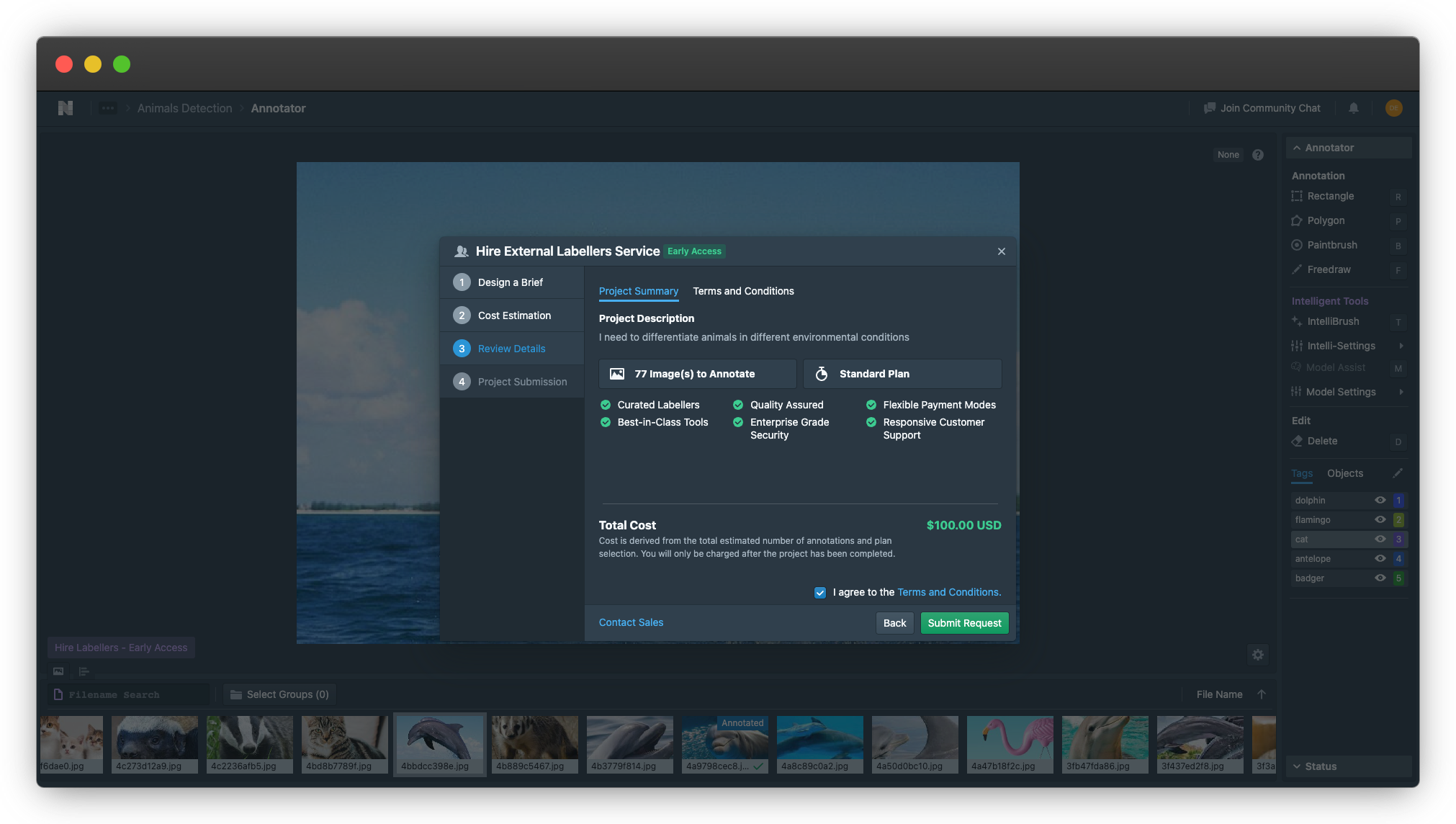
When the annotation job is confirmed, the external labelling services will gain access to your project and will be allowed to annotate on your behalf. So as to reduce confusion, you will not be able to edit or make your own annotations while the annotation job is being completed. Using our Asset Group Management, you can upload images under groups so that the external annotators will only annotate certain types of images. The external annotators will also be able to benefit from our top-of-the-line annotator tools which will help to produce efficient and high quality annotations.
Step 4: Review Scheduled Jobs
To see previous jobs that you have scheduled, you can select Scheduled Jobs on the sidebar of the Nexus homepage. There, you will be able to see all jobs, completed and currently pending. For each job, there will be a progress bar showing the annotation job’s level of completion, as well as a status at the top right to show whether it is pending or completed. In the additional settings found at the bottom right, you can export your brief which will show the overview of the request in PDF, mark the job as completed, or contact the team for additional help.
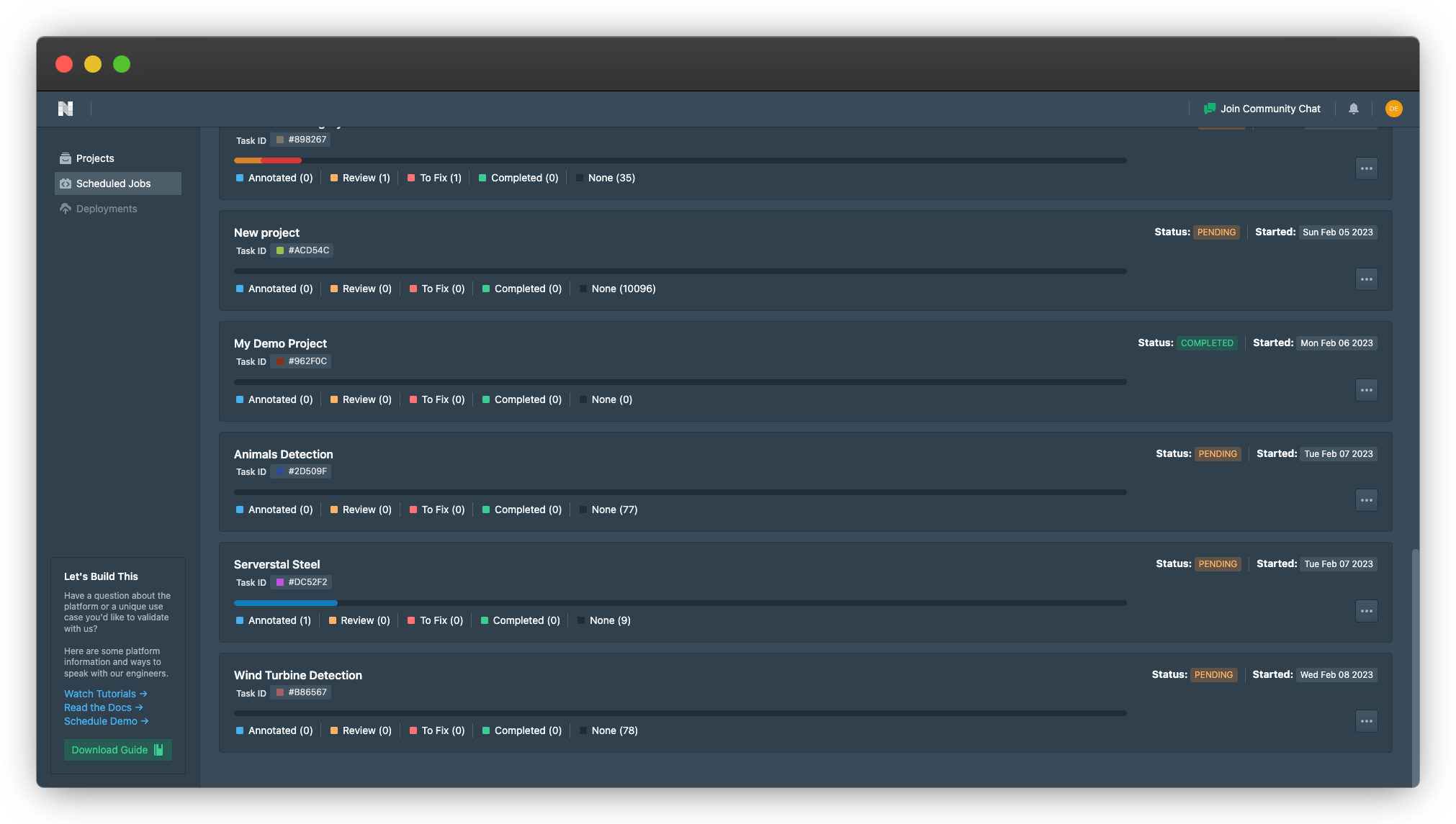
When the annotation job is finished, you will regain full access to your project and start to build your ideal workflow, and train your computer vision model!
Is Your Data Secure on Nexus?
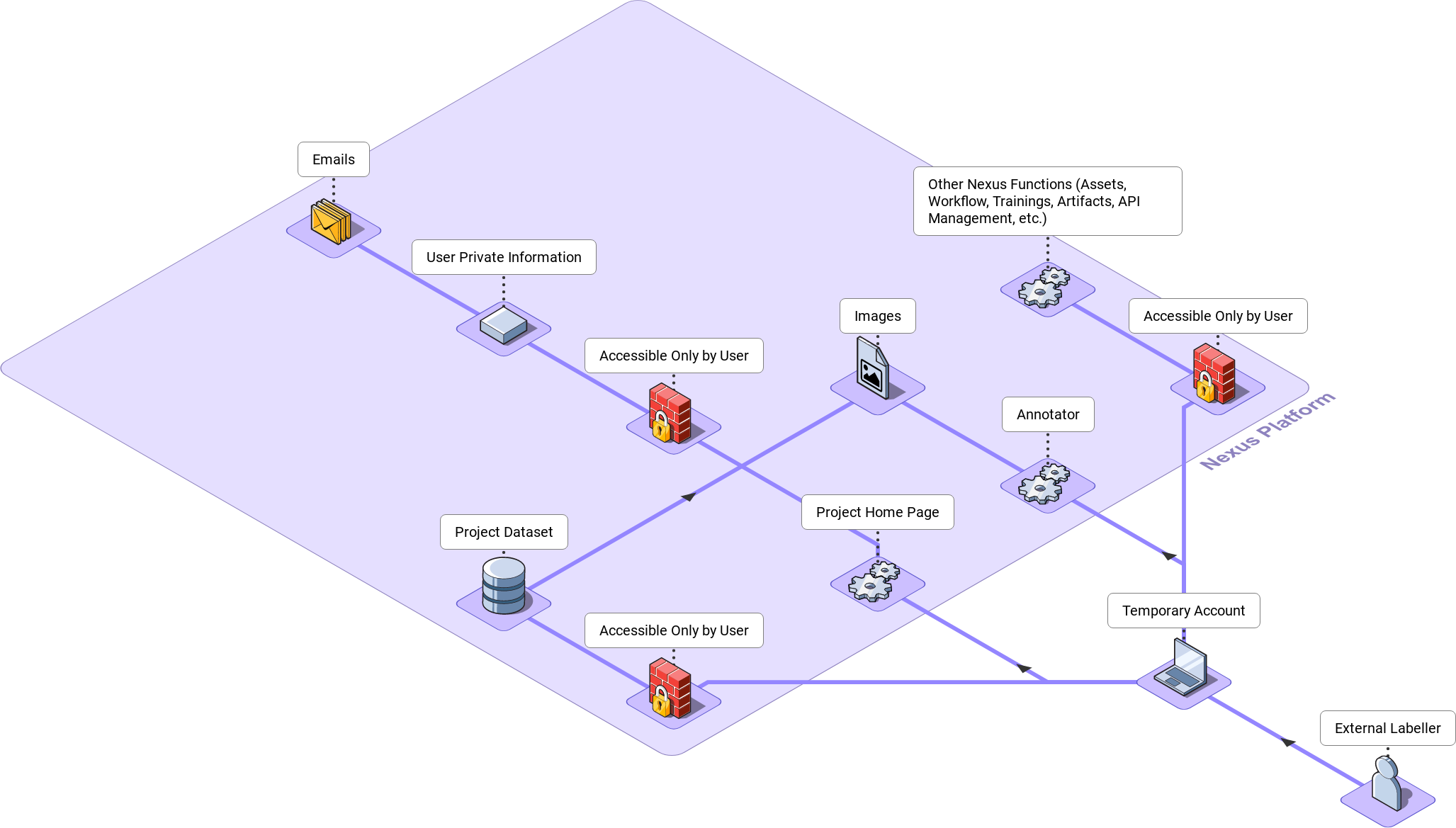
At Datature, we work tirelessly to maintain the highest standards of data security. To maintain data integrity for our external labeller infrastructure, we support this in three main ways. Firstly, during a job, an external labeller is provided a temporary account with limited access to only the project homepage and the annotator. Secondly, private information specific to the user that may be visible on the homepage such as emails on the Collaborators tab will be removed for these external labeller accounts, thus preserving the private user information. Finally, external labellers on the Annotator page will only have access to the images that were assigned to them via the labelling request, and will not have any ability to access other images in the same project dataset. Overall, Nexus keeps user data private, restricts the scope of the dataset access to relevant assets, and limits feature access to just the Annotator and broad project statistics.
What Factors Affect the External Labelling Cost?
There are a few dimensions that are used to calculate the cost of a task: difficulty, scale, and speed. Difficulty covers the difficulty of the annotation task. Segmentation masks are much more difficult to annotate well, and are much more time consuming to annotate for the labeller as compared to bounding boxes. Scale considers the average number of annotations per image as well as the number of images needed for annotation. Based on the sample annotations, we try to estimate the total number of annotations needed and base the cost on this. Finally, speed plays a factor in terms of how many labellers will be needed to label the dataset given a certain pace or deadline.
Next Steps
Now that you are having your assets labelled by a team of expert external labellers, you are now free to look at what model you want to train. Check out this article about training your model on a popular offshoot of the YOLO model series, YOLOX, to see how to conduct your own model trainings.
Need Help?
If you have questions, feel free to join our Community Slack to post your questions or contact us about how the External Labelling Service fits in with your usage.
For more detailed information about the External Labelling functionality, customization options, or answers to any common questions you might have, read more about the annotation process on our Developer Portal.
Build models with the best tools.
develop ml models in minutes with datature