The new SAM-2 Model
Meta has taken computer vision to the next level with the release of the Segment Anything Model 2 (SAM 2). Building on the success of the first model, SAM 2 brings amazing new features for segmenting images and videos. Whether you're in the creative industry, medical field, or working on self-driving cars, SAM 2 is here to transform your work. Read on to find out everything about this new technology and how Datature is using it to provide top-notch AI solutions.
Understanding Segmentation
Image segmentation is a key task in computer vision that involves figuring out which parts of an image belong to specific objects. This is important for many applications, from medical imaging and self-driving cars to photo editing and augmented reality. Traditional methods often needed a lot of manual work and were limited to specific tasks or datasets.
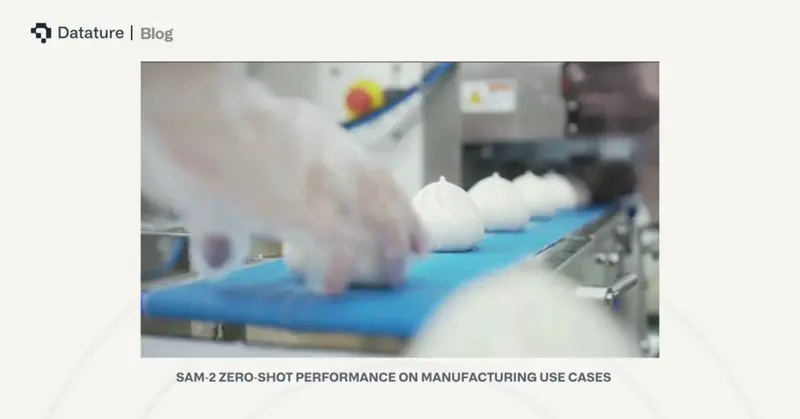
The Original Segment Anything Model
Released in April 2023, the first Segment Anything Model (SAM) aimed to make image segmentation easier for everyone. It provided a flexible model that could handle many different tasks without needing specific training data for each task. SAM introduced several key features:
- Promptable Interface: SAM could be guided by different prompts, like clicks, boxes around objects, or text, to create segmentation masks.
- Zero-Shot Transfer: SAM could understand new tasks and image types without additional training, a feature known as zero-shot transfer.
- Large-Scale Dataset: SAM was trained on a massive dataset, the Segment Anything 1-Billion mask dataset (SA-1B), which included over 1 billion segmentation masks from diverse images.
These features made SAM versatile for many applications.
What’s New in SAM 2?
SAM 2 builds on the original model with several exciting improvements:
- Enhanced Accuracy and Speed: SAM 2 offers better segmentation accuracy and faster processing times, making it great for real-time use.
- Video Segmentation: SAM 2 can now segment videos, tracking and segmenting objects across video frames consistently.
- Refined Prompting Mechanisms: The new model supports more advanced prompting techniques, giving users more control over the segmentation process

- Expanded Dataset: SAM 2 is trained on an even larger and more diverse dataset, improving its ability to handle different image and video types
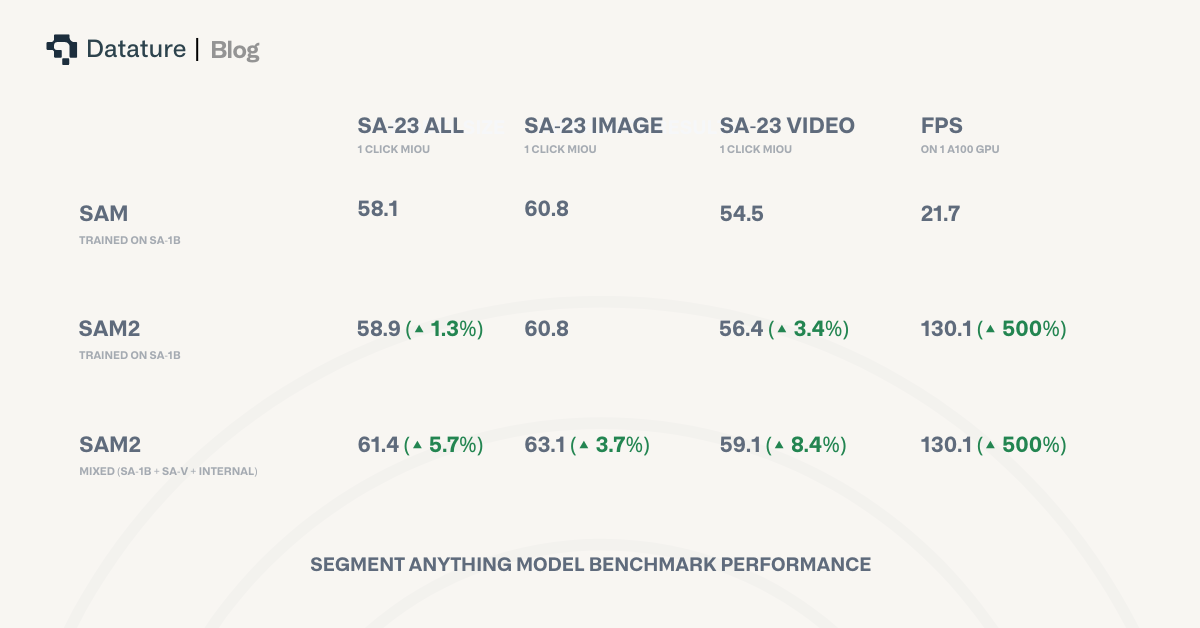
How SAM 2 Works
Image Encoder
SAM 2 uses a simple model architecture which uses a pre-trained Hiera (hierarchical) image encoder to consume frames one at a time. This hierarchical encoder allows for the use of multi-scale features, which are crucial for capturing details at different levels of resolution during the decoding process.

Memory Attention
These features are then passed to a memory attention module which leverages self-attention and cross-attention to condition the current frame’s features on the previous frames’ features and predictions. Initially, with an empty memory bank, cross-attention of spatial feature maps and semantic information with the current frame’s features cannot be done. However, as SAM 2 gains spatio-temporal knowledge, the spatial feature maps and semantic information stored in the memory bank are used to cross-attend the current frame’s features to the previous frames’ features and predictions. Thus, the attention mechanism now has the ability to leverage knowledge from the past to make new predictions on the present.
Prompt Encoder and Mask Decoder
The original prompt encoder from the original SAM is used to encode mask, points, and box prompts which help define the boundaries of a given object. The mask decoder is heavily influenced by SAM’s mask decoder except that SAM 2’s mask decoder allows for no valid mask to exist in a frame whereas the original SAM requires there be a mask in a given frame (image), which allows for SAM 2 to robustly handle object occlusion. The mask decoder then outputs a predicted mask on the current frame.
Memory Encoder
The memory encoder then downsamples the predicted mask and sums it together with the multi-scale features of the current frame. Subsequent lightweight convolutional layers help to fuse this information and form a “memory”. This supplies the model with information from past predictions and frames allowing SAM 2 the potential to understand spatio-temporal relationships in the supplied video.
Memory Bank
The memory bank is used to retain memories about recent frames and previously prompted frames which are both stored as spatial feature maps, where the recent frames also embed temporal position information to encode short-term object motion – improving SAM 2’s object tracking capabilities. Moreover, semantic information is also stored about the segmented objects and is based on the output of the mask decoder.

Applications of SAM 2
Creative Industries
- Improve video editing, create unique visual effects, and control generative video models with precision object control.
Medical Imaging and Scientific Research
- Enhance accuracy in identifying anatomical structures and support scientific studies with precise segmentation.
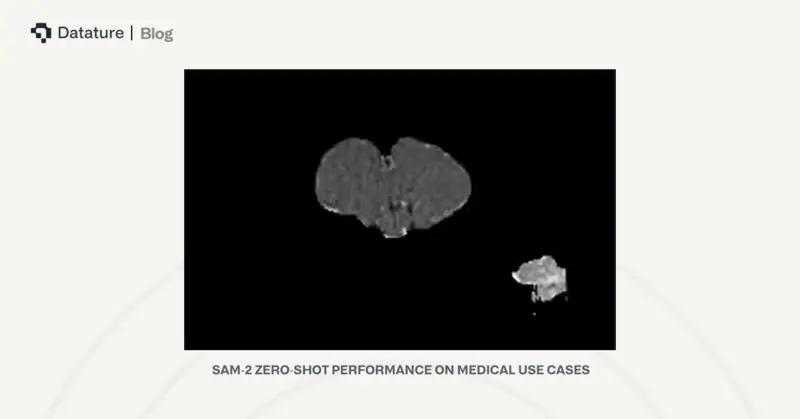
Autonomous Vehicles
- Boost perception capabilities and ensure better navigation and obstacle avoidance in self-driving systems.
Data Annotation
- Speed up the creation of annotated datasets, reducing manual annotation time and effort.
Start Using SAM 2 with Datature!
Datature is excited to integrate Meta's SAM 2 into our Nexus platform. This integration will provide users with advanced object segmentation capabilities, whether working with images or videos.
Benefits of Using Datature with SAM 2:
- Easy Integration: Incorporate SAM 2 into your projects without needing extensive technical expertise.
- Accelerated Annotation: Speed up tedious annotation tasks by automating the segmentation process, eliminating the need for full manual labeling of individual images and video frames.
- Scalability: Handle large datasets and scale your segmentation tasks effortlessly.
- Customizability: Adjust the segmentation process with flexible prompting options and adjustable parameters.
Transform Your Projects with Datature
Meta’s Segment Anything Model 2 represents a huge step forward in computer vision, offering enhanced capabilities for both image and video segmentation. By integrating SAM 2 with Datature, you can take full advantage of this powerful technology to streamline your workflows and achieve superior results in your projects.
Ready to revolutionize your computer vision projects? Experience the future of image and video segmentation with Datature. Book a demo today and discover how our platform can elevate your AI capabilities to new heights. Don’t miss out on the opportunity to harness the power of the latest advancements in AI. Visit Datature to get started.
Build models with the best tools.
develop ml models in minutes with datature